Nick Seaver's Computing Taste: Algorithms and the Makers of Music Recommendation is a book about how modern infrastructure is built, and how its builders see it. It is a book steeped in the history of technology, and it seeks to understand the social and cultural motivations behind that history. In this it is successful — and along the way, Seaver manages to establish a compelling intellectual environment and proposes a string of thought-provoking philosophical discussions.
Nick Seaver joined Scope of Work's Members' Reading Group recently to discuss the book; below is an edited and abbreviated transcript of our conversation.
Spencer Wright: Nick, I would love to start by asking how you listen to music. Which you don't really get into much, in this 200-plus-page book about the systems via which we listen to music.
Nick Seaver: So how do I listen to music? I'll say that it's changed for me, largely as a result of having kids, which is normal. In my Spotify Wrapped last year, when they gave you the types of listener you are, I got the robot one, the one that just listens to the recommendations, which I felt was especially damning. But apparently, I listen to whatever the Spotify algorithm tells me to listen to these days. It was different [when I wrote Computing Taste]: I will say that while I was doing the research for this book, I was very much a SoundCloud, new music kind of listener, as were most of the people who I was talking to who are in the book. But now, if I go back to my SoundCloud, I can see in my likes history this period of time, and that brings me immediately back to when I was doing field work.
SW: Do you do that often? Or are you just like, "I'm happy to be the Spotify robot guy?"
NS: I was just doing this, actually, this week. It comes and goes. But I was digging through my likes history in Spotify, cause you get into these local minima, and it keeps recommending the same things to you over and over, and that's the bummer of trying to be the robot. At some point, I feel like I tried to use my likes history to replicate an old iTunes library I had. I feel like I have a very poor memory for these things spontaneously, so if I'd find something, it's like, "Oh right, I used to listen to this kind of music all the time, and I haven't thought about it at all."
SW: I think your bio on your website says something about how you study how people use technology to make sense of cultural things. What does that mean in the context of music recommendation algorithms?
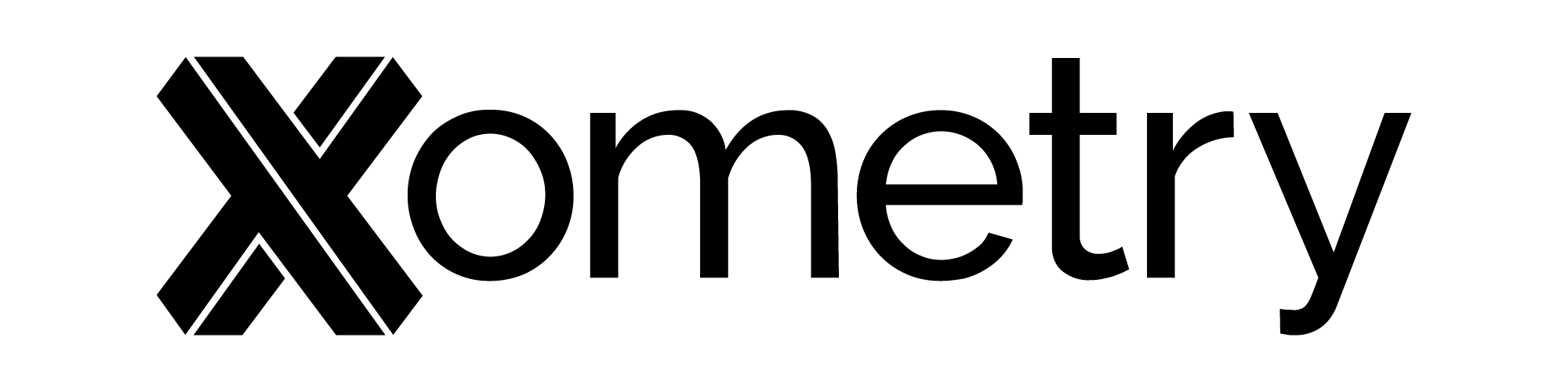
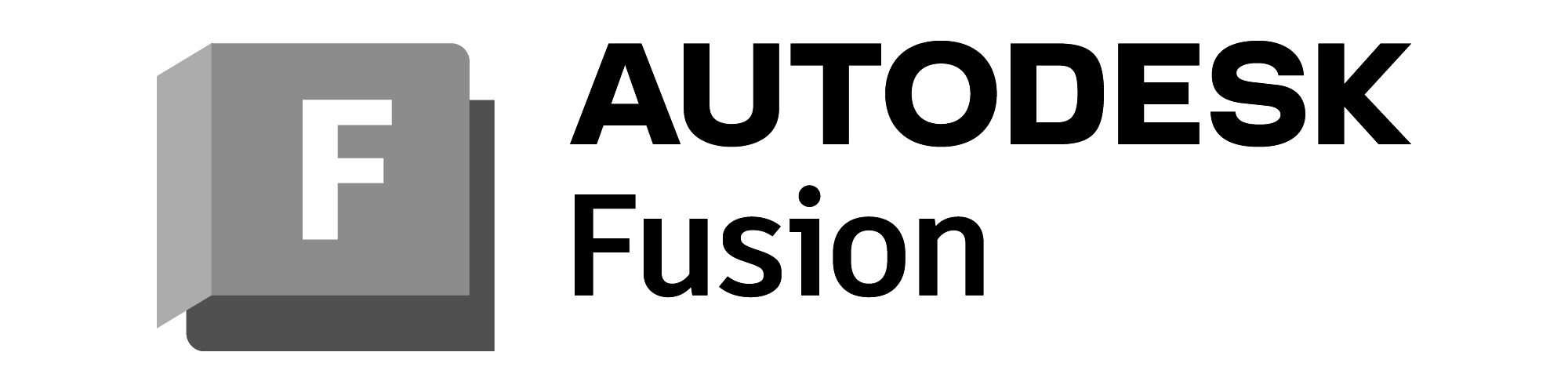
NS: So the line I usually give is, one of the fields I'm associated with is called Science and Technology Studies, STS. And the usual move that people in my field do is we find something that people think is not cultural and talk about how it actually is cultural. A classic example of this would be road infrastructure. People are like, "Well, road infrastructure's about engineering, it's about where the roads should go, obviously," and we say, "Well, actually, there's also the cultural stuff that goes into how you design roads, bridges, decide where they go, what size they should be, and so on." It’s a little bit of a gotcha move that we use. And what I thought was interesting about studying recommender systems was that you can't do that trick, because everybody who works on music recommender systems knows that they're cultural. They know that it's not objective.
And so I'm interested in what they do with that information, how it changes the way that they think. Because once we point out how something is cultural, say AI and bias, for instance, then that will lead to some sort of change, that will lead to some difference in how people do what they do. So I'm very interested in how people deal with the fact that [music recommender] technology and culture are always intertwined with each other.
SW: Why was music the thing that you chose to highlight this?
NS: I have a very arbitrary reason for this, which is fun. So [chuckle] this was based on my doctoral dissertation in Anthropology. When I was applying to PhD programs, I was applying as someone who was doing a Master's degree in Media Studies at MIT (which is not the media lab; that’s a different thing). My thesis was on the history of the player piano, and so I was really interested in automation and music, which is, again, one of those spots where technology and culture intertwine. We think of music as being cultural, human-expressive, but you can't make music or listen to music without a ton of technology, and a piano is a big, old machine. It's a music-making machine.
I was interested in how people deal with that tension. We have this idea that technology should be rational and objective, and so on, and music and other cultural things are the opposite of that, and yet they're always together. So I was applying to an anthropology program, and I was like, "I wanna study something that's about music and automation, but there's not really a lot of player piano stuff going on now, so what else exists?” Well, recommender systems exist. I applied with that project, and sort of unusually, I did not change it from then. The algorithm stuff came after that; in my field, there's a lot of work on algorithms now, and it's a hot topic, and I got into it for the least hot reasons.
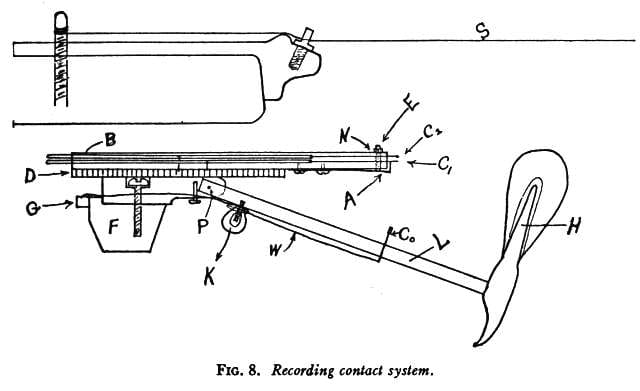
SW: Can you tell us more about the history of player pianos? I'm so curious.
NS: What are the fun things… So my first academic publication, an article I wrote, actually, is an article on the history of the player piano. One thing in the history of audio reproduction is how the idea of fidelity is established. We have an ordinary idea that fidelity just means how accurate a recording is, but people care about specific things getting reproduced in an audio recording; this is the social construction of fidelity. There's a great book called The Audible Past by Jonathan Sterne, which is about this for phonographs, and with the player piano, there's this fantasy that you can have perfect reproduction, because the piano's a big machine, you press the buttons on it at certain times with certain forces, and that, in theory, should get you everything. So it should be possible to reproduce a piano performance exactly based on that.
It turns out it's not that straightforward, and people did a lot of work to try to say that their recordings were the same as the original performance. But there's this whole funny transmutation of, like, "How do you know how hard to hit the key?" When you hit a piano key, it throws a hammer at the strings. This is a ballistics situation, because nothing is touching the hammer on its way there. So there's a guy [who happens to also have invented the bazooka!] who develops a technique for measuring the speed of the hammer. You put one wire on the hammer, and two stationary wires next to it, and as the hammer moves, its wire touches the stationary wires, completing a circuit each time and drawing little dots on a piece of paper. You measure the distance between the dots, and that tells you how fast the hammer is going.
The one other factoid that I just love is that the player piano and the phonograph were invented within two years of each other. Today, we think of the player piano being really old and being obsoleted by a real reproduction technology — the phonograph. But the pianola, which is the first player piano, was invented just two years before the phonograph. And the line people will give you in media history is that if you ask a guy on the street, circa 1900, which of these two technologies was a sort of a gimmick, and which of them was a legit way of recording music, they would say the phonograph was a gimmick and the player piano was the real thing. That was so fascinating to me, to kind of recover that old common sense.
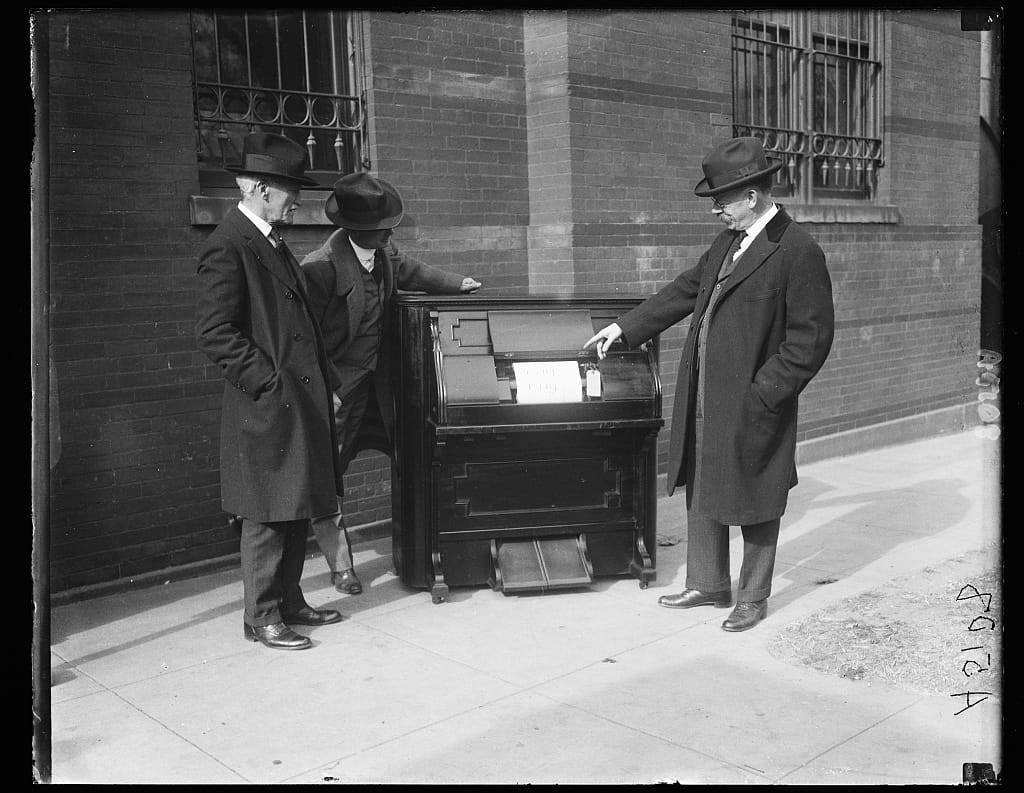
SW: Wait, so a player piano uses a roll of paper to transcribe what’s played, right?
NS: Yeah, it's a roll of paper with holes in it that runs over a vacuum. And when the vacuum hits a hole, then there's a variety of contractions that turn that pressure differential into enough power to fling the hammer. There are a bunch of different ways of doing that, it turns out. There's binary code going on, so there's usually 64 dynamic levels, because you have basically an array of pumps that you can use in multiples of two.
SW: And the whole thing is actually created by somebody playing a song on a piano?
NS: Sometimes. Sometimes they're punched out by hand, from a score. Sometimes they're recorded and there's a separate set of technologies to do that. But even when they're recorded, there's this kind of hand fudging of it. Sometimes the dynamic levels are recorded automatically, sometimes they're not. There's a German company that tried to make a system to capture continuous variation in dynamic level.
But the other fun thing is that if you were a person who had a player piano at home, you could control the speed, and you could control the dynamic level. I went to this archive, the International Piano Archives at Maryland, which has this great collection of marketing materials from player piano companies around the turn of the century. And they were trying to encourage people to imagine that the expressive part of music was actually not what the notes were, but how loud you played them and how you sped up and slowed down. So the idea was that the player piano companies had removed all of the drudgery of piano-playing, pressing the right notes, and then gave you the extracted musical part. So you, the owner of the player piano, just get to be expressive on top of this.
There's actually a lot of connections to contemporary AI art stuff, like the idea that all of the drudgery of actually knowing how to paint, or whatever, is dumb. The creative part is Pikachu riding a horse or whatever, coming up with the idea of the thing.
SW: This is all amazing — and also we should probably ask you questions about Computing Taste. In the book you spent a summer interning at a company that you call Whisper. What were you doing there? Like were you an actual intern? And then, how did your coworkers engage with your role there?
NS: Yes! So I was, like, a fake intern. At the time, Whisper had two kinds of interns. This has been fairly common in a lot of tech companies: They had the computer science interns who got, like, handsomely paid and worked on little projects. And they had business interns who were usually music students and who did not get paid, did grunt work, and were just glad to be there. I was sort of the latter, except that I had been brought in by someone who was — he was someone who would often be called a lone wolf coder. He was like that guy, who had been at the company from the beginning, and who did sort of lead gen, weird projects. And, like, nobody could really be his boss, but companies keep people like this around until they get big enough that someone says “who the fuck is this guy?” But in the meantime everyone sort of respects him. Anyway, I had tried to get into this company before and did not succeed, but I had met this guy, and he invited me in, and after that everyone said “oh, you're with him? Yeah, you're fine.” So I was his intern. He didn't normally have interns, and I didn't really have to do anything. So I got to float around a lot and I did a ton of interviews. I wanna say there were like 70 to 80 people in that company, and I probably interviewed about 60 of them in the course of the time that I was there
Separately, I was going to conferences where there were academic and industry people working and talking to people there. In those contexts, people are actually talking to each other: They're sort of sharing things they're not supposed to be sharing. And that was almost more useful because I ended up focusing on the sector rather than on one specific company.
SW: This sounds a little bit like the anecdote in the epilogue, in which one interviewee basically said that corporate anthropology is bullshit.
NS: Yes, Yes.
SW: I think when we discussed this part of the book, we all kind of thought it was a straw man. Like, corporations are just like any other entity you could study, and just as you could understand a village in the Amazon, by going there and talking to the people, you could also understand a startup office in San Francisco.
NS: I agree with you. I think that there is an idea that companies, or people in the West, are uniquely dissembling: We lie more, and we are harder to get a hold on. But people lie everywhere. I have to thank my academic mentors for this, because anytime I would claim that I had some unique problem, because I was studying companies or algorithmic system development, they'd be like “that's not unique. We have this problem too. There are secret societies in the places that we study.”
SW: To what extent did the people that you spoke with during this research follow what you were publishing?
NS: I wouldn’t say they followed me that closely. I did get a lovely message from one of the people who was in the book under a pseudonym, and he read it before I even got my copy. Like he bought his copy, and I hadn't even gotten my author copy yet, and he had read it over the weekend. And he was like, “I think this was fair. There were some bits where I felt criticized, but looking back on it (and this is the nice out that you get from academic timelines), well, that was how we thought, you know, years ago.” But most of the people I interviewed didn’t care about the book.
One funny thing you have as an anthropologist is that your job is sort of to tell one group of people what another group of people think, and to sort of add your own interpretive layers on top of it. And what's funny about the group that I studied [people involved with tech companies], is that those people talk a lot, and they talk a lot in public, and people listen to them.
SW: Right, right. Marc Andreessen [famous venture investor, who is quoted in Computing Taste] is out there tweeting regardless of whether you're re-quoting him.
NS: He will never stop tweeting. Historically, anthropologists study people who are disadvantaged, and we do so enough that when the American Anthropological Association revised their ethics statement, maybe 10 years ago, they said that anthropologists had an obligation to help the people that they study. And all the anthropologists like me, and my friend Daniel Souleles, who writes about private equity investors, are like, “are you serious? I should not help private equity investors do what they're doing. That is not my obligation. They've helped themselves plenty.”
Jay Williams: This reminds me of early in the book, where you write about this whole strain of anthropology that claims that algorithms are impossible to know about. They’re portrayed as this huge black box, and only this weird wizard-like caste knows anything about it. But the people who work on these algorithms talk about them constantly. You can go to Stack Overflow and find out how all of these algorithms work. You can buy an O'Reilly book. It is the most see-through black box I've ever heard of in my life.
NS: Yeah, I think that was part of the appeal for me — just being able to do ethnographic research of this stuff, because you can just go talk to people and they will talk to you. It is not all secrets. I think the black box is an abused concept. It started as a technique to ignore something’s internal complexity, but it came to mean that something has been kept secret on purpose. And therefore the black box becomes part of the history of corporate legal secrecy, which means that our idea about what an algorithm is gets packaged up with ideas about how corporations work. I talk a bit about this in the book; it's a corporate structure more than it is a technical computational structure.
SW: I wonder if you could just talk a little bit about your recent work on attention.
NS: I'm interested in how attention has come to appear as the solution to, or the explainer of, like, all of our problems. So I've done a bunch of interviews with computer scientists, and I went to NeurIPS to study deep learning scientists who are interested in attention. Anyway, what I'm working on during my current sabbatical, is a book about the multiplicity of what we talk about when we talk about attention. Attention seems to be really obvious. We all know what it is. It's that thing where you grab onto something with your mind. But it turns out that we mean a ton of things by attention. It means how long you can stay put; it means your ability to filter out the environment; it means mental energy; it means caring; it means your ability to cognize things. If you buy a car with any sort of self-driving feature in the US or Europe, it is by regulation required to have a driver attention system in it. If you've used a car that has any of these, you know there are many ways to do it: pressure on the steering wheel, cameras pointed at you to see if you're looking at the road. These are used for truck drivers, for instance, to see whether they are falling asleep or not. (Karen Levy’s Data Driven: Truckers, Technology, and the New Workplace Surveillance has a chapter about this — it’s so good!)
I did some field work for this book, which will be called Attention Fragments, with online survey designers. So, you know, if you take an online survey, there will be trick questions in the survey to make sure you're paying attention. This is so interesting, because they use a really weird definition of attention. It's not how long you’re watching something, or whether you’re looking at it, but, are you understanding the questions? So it'll be, you know, a trick question that has long instructions in the middle, telling you that in the next question, answer X, no matter what the real answer is. And then surveyors will use those questions to kick out results.
The problem is that if you do that, you're kicking out results not at random. And the survey designers want to keep as much stuff in the survey as possible. So you have these new techniques for adding lots of those questions and not kicking out any results at all, but instead adding an attention variable to their model to explain their results, which is weird because you're like, I don't want to kick these results out. And yet the data I'm collecting seem to indicate that these people are not reading the questions. So like, what does that mean?
SW: It’s as if Van Halen really wants to play the show, but the brown M&Ms are still in the bowl.
NS: The one last thing I'll say is about eye tracking. People often think of eye tracking as being the real measure of attention. Everything else is a proxy for eye tracking. But eye tracking is also a proxy for attention. If you've ever had to look at eye tracking data, you know that it's bizarre: People are just looking all over the place, all the time. And you never know whether they're paying attention to the thing or not. At the end of the day, you can look right at something and maybe not be paying attention to it. So all of these measurement techniques bump up against the sort of fundamental impermeability of the head; basically, we don't know what attention is.
Thanks so much to Nick Seaver for joining us! And thanks to our Members and Supporters for making this newsletter possible.